I just read this super interesting article about student evaluations of teaching as a “technology of power” that acts to prevent any change of the system from the status quo, that I can’t stop thinking about.
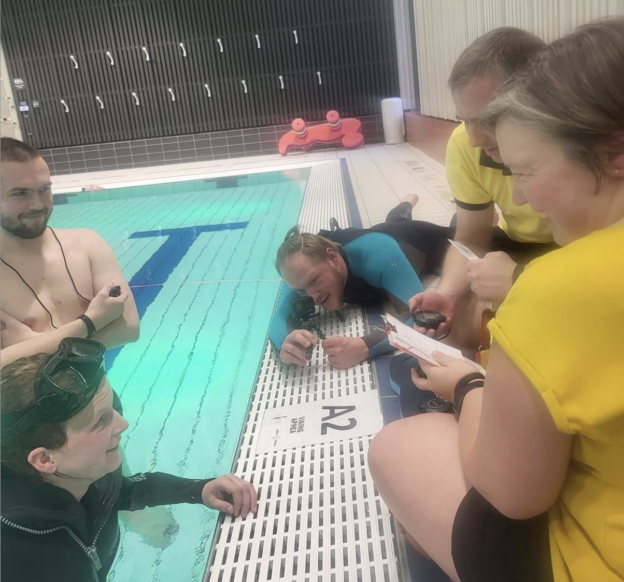
I just read this super interesting article about student evaluations of teaching as a “technology of power” that acts to prevent any change of the system from the status quo, that I can’t stop thinking about.
There are a lot of problems with student evaluations of teaching, especially when they are used as a tool without reflecting on what they can and cannot be used for. Heffernan (2021) finds them to be sexist, racist, prejudiced and biased (my summary of Heffernan (2021) here). There are many more factors that influence whether or not students “like” courses, for example whether they have prior interested in the topic — Uttl et al. (2013) investigate the interest in a quantitative vs non-quantitative course at a psychology department and find a difference in interest of nearly six standard deviations! Even the weather on the day a questionnaire is submitted (Braga et al., 2014), or the “availability of cookies during course sessions” (Hessler et al., 2018) can influence student assessment of teaching. So it is not surprising that in a meta-analysis, Uttl et al. (2017) find “no significant correlations between the [student evaluations of teaching] ratings and learning” and they conclude that “institutions focused on student learning and career success may want to abandon [student evaluation of teaching] ratings as a measure of faculty’s teaching effectiveness”.
But just because student evaluations of teaching might not be a good tool for summative assessment of quality, especially when used out of context, that does not mean they can’t be a useful tool for formative purposes. Roxå et al. (2021) argue that the problem is not the data in itself, but the way it is used, and suggest using them — as academics do every day with all kinds of data — as basis for a critical discourse, as a tool to drive improvement of teaching. They suggest also changing the terminology from “student rating of teaching” to “course evaluations”, to move the focus away from pretending to be able to measure quality of teaching, towards focussing on improving teaching.
In that 2021 article, Roxå et al. present different way to think about course evaluations, supported by a case study from the Faculty of Engineering at Lund University (LTH; which is where I work now! :-)). At LTH, the credo is that “more and better conversations” will lead to better results — in the context of the Roxå et al. (2021) article meaning that more and better conversations between students and teachers will lead to better learning. “Better” conversations are deliberate, evidence-based and informed by literature.
At LTH, the backbone for those more and better conversations are standardised course evaluations run at the end of every course. The evaluations are done using a standard tool, the “course experience questionnaire”, which focusses on the elements of teaching and learning that students can evaluate: their own experiences, for example if they perceived goals as clearly defined, or if help was provided. It is LTH policy that results of those surveys cannot influence career progressions; however, a critical reflection on the results is expected, and a structured discussion format has been established to support this:
The results from those surveys are compiled into a working report that includes the statistics and any free-text comments that an independent student deemed appropriate. This report is discussed in a 30-45 min lunch meeting between the teacher, two students, and the program coordinator. Students are recruited and trained specifically for their role in those meetings by the student union.
After the meeting and informed by it, each of the three parties independently writes a response to the student ratings, including which next steps should be taken. These three responses together with the statistics then form the official report that is being shared with all students from the class.
The discourse and reflection that is kick-started with the course evaluations, structured discussions and reporting is taken further by pedagogical trainings. At LTH, 200 hours of training are required for employment or within the first 2 years, and all courses include creating a written artefact (and often this needs to be discussed with critical friends from participants’ departments before submission) with the purpose of make arguments about teaching and learning public in a scholarly report, contributing to institutional learning. LTH also rewards excellence in teaching, which is not measured by results of evaluations, but the developments that can be documented based on scholarly engagement with teaching, as evidenced for example by critical reflection of evaluation results.
At LTH, the combination of carefully choosing an instrument to measure student experiences, and then applying it, and using the data, in a deliberate manner has led to a consistent increase of student evaluations of the last decades. Of course, formative feedback happening throughout the courses pretty much all the time will also have contributed. This is something I am wondering about right now, actually: What is the influence of, say, consistently done “continue, start, stop” feedbacks as compared to the formalized surveys and discussions around them? My gut feeling is that those tiny, incremental changes will sum up over time and I am actually curious if there is a way to separate their influence to understand their impact. But that won’t happen in this blogpost, and it also doesn’t matter very much: it shouldn’t be an “either, or”, but an “and”!
What do you think? How are you using course evaluations and formative feedback?
Braga, M., Paccagnella, M., & Pellizzari, M. (2014). Evaluating students’ evaluations of professors. Economics of Education Review, 41, 71-88.
Heffernan, T. (2021). Sexism, racism, prejudice, and bias: a literature review and synthesis of research surrounding student evaluations of courses and teaching. Assessment & Evaluation in Higher Education, 1-11.
Hessler, M., Pöpping, D. M., Hollstein, H., Ohlenburg, H., Arnemann, P. H., Massoth, C., … & Wenk, M. (2018). Availability of cookies during an academic course session affects evaluation of teaching. Medical Education, 52(10), 1064-1072.
Roxå, T., Ahmad, A., Barrington, J., Van Maaren, J., & Cassidy, R. (2021). Reconceptualizing student ratings of teaching to support quality discourse on student learning: a systems perspective. Higher Education, 83(1), 35-55.
Uttl, B., White, C. A., & Morin, A. (2013). The numbers tell it all: students don’t like numbers!. PloS one, 8(12), e83443.
Uttl, B., White, C. A., & Gonzalez, D. W. (2017). Meta-analysis of faculty’s teaching effectiveness: Student evaluation of teaching ratings and student learning are not related. Studies in Educational Evaluation, 54, 22-42.
More methods today, inspired by E.-M. Schumacher’s “Methoden 2 go online!“! Today:
I used to hate it when in in-person workshops everybody was asked to give a statement at the end, about what the most important thing was they learned, or how they liked something, or that kind of thing because on the pressure I felt in those situations. But virtually, fo example as a lightening storm in the chat, I rather like the method because it gives an equal voice to everybody instead of a few people dominating everything, and it’s also documented rather than just everybody just quickly saying something before then rushing off. It’s definitely a nice way to get a quick impression from everybody!
Doing this synchronously (as in everybody submitting what they wrote at the same time) also gives you an overview that is less biased as in there wasn’t some kind of group opinion forming as people started talking, that other people later did not want to go against. And sometimes there are weird group dynamics at play when people start off negatively and everybody just keeps piling on…
Another method I quite like: asking students to write a letter to themselves where they reflect on what they learned. This can happen virtually as an email, and I’ve even used it in in-person workshops on paper, where people then put it in a sealed envelope and we sent it out to them a couple of weeks later. I really liked getting those letters from former me, especially when I had set goals or points to follow up on, and was reminded of them! The time delay there is quite useful (spaced repetition? ;-)) and also getting hand-written mail (even if written by myself) is always nice…
Five finger feedback can be done in in-person workshops, but also virtually (for example in a table with five columns where everybody notes down their comments).
1) The thumb. What went well? 2) The index finger. What could be improved? 3) The middle finger. What went wrong? Negative feedback. 4) The ring finger. What would we like to keep? 5) The pinkie finger. What did not get enough attention?
In in-person settings, this tends to take a looong time, and also put too much pressure on participants to make me feel comfortable, but I can see this working a lot better online!
This is another fun method to look at what students want to remember from a lesson: Having a graphic of a suitcase or bag, and then adding sticky notes with the things students want to take away from the workshop. Works offline as well as online! But then it’s not really different from minute papers etc, so maybe use it to spice things up occasionally. Or, if you use it regularly, seeing the graphic of the luggage might already act as trigger for students so they start on the task, without you having to remind them. That might actually also work well!
Always a great method: Asking students to come up with good exam questions. They can then be discussed in small groups or with the large group, used as exercises practicing for the exam, or even used in the final exam!
But beware: Coming up with good exam questions is really difficult and students might need a lot of guidance, for example discussing a grading rubric and what kind of knowledge and skill should be able to be shown by completing an exam question. And I would always also ask them to provide the solution with the question, otherwise it is really difficult for students to get a good idea of how difficult or easy a question is (usually they become super difficult if students try to make them interesting).
That’s it for now about E.-M. Schumacher’s “Methoden 2 go online!“! There are plenty more where these came from, would you be interested in reading about more?
One of my pet peeves are student evaluations that are interpreted way beyond what they can actually tell us. It might be people not considering sample sizes when looking at statistics (“66,6% of students hated your class!”, “Yes, 2 out of 3 responses out of 20 students said something negative”), or not understanding that student responses to certain questions don’t tell us “objective truths” (“I learned much more from the instructor who let me just sit and listen rather than actively engaging me” (see here)). I blogged previously about a couple of articles on the subject of biases in student evaluations, which were then basically a collection of all the scary things I had read, but in no way a comprehensive overview. Therefore I was super excited when I came a sytematic review of the literature this morning. And let me tell you, looking at the literature systematically did not improve things!
In the article “Sexism, racism, prejudice, and bias: a literature review and synthesis of research surrounding student evaluations of courses and teaching.” (2021), Troy Heffernan reports on a systematic analysis of the existing literature of the last 30 years represented in the major databases, published in peer-reviewed English journals or books, and containing relevant terms like “student evaluations” in their titles, abstracts or keywords. This resulted in 136 publications being included in the study, plus an initial 47 that were found in the references of the other articles and deemed relevant.
The conclusion of the article is clear: Student evaluations of teaching are biased depending on who the students are that are evaluating, depending on the instructor’s person and prejudices that are related to characteristics they display, depending on the actual course being evaluated, and depending on many more factors not related to the instructor or what is going on in their class. Student evaluations of teaching are therefore not a tool that should be used to determine teaching quality, or to base hiring or promotion decisions on. Additionally, those groups that are already disadvantaged in their evaluation results because of personal characteristics that students are biased against, also receive abusive comments in student evaluations that are harmful to their mental health and wellbeing, which should be reason enough to change the system.
Here is a brief overview over what I consider the main points of the article:
According to the studies compiled in the article, your course is evaluated differently depending on who the students are that are evaluating it. Female students evaluate on average 2% more positively than male students. The average evaluation improves by up to 6% when given by international students, older students, external students or students with better grades.
It also depends on what course you are teaching: STEM courses are on average evaluated less positively than courses in the social sciences and humanities. And comparing quantitative and qualitative subjects, it turns out that subjects that have a right or wrong answer are also evaluated less positively than courses where the grades are more subjective, e.g. using essays for assessment.
Additionally, student evaluations of teaching depend on even more factors beside course content and effectiveness, for example class size and general campus-related things like how clean the university is, whether there are good food options available to students, what the room setup is like, how easy to use course websites and admission processes are.
Many studies show that gender, ethnicity, sexual identity, and other factors have a large influence on student evaluations of teaching.
Women (or instructors wrongly perceived as female, for example by a name or avatar) are rated more negatively than men and, no matter the factual basis, receive worse ratings at objective measures like turnaround time of essays. Also the way students react to their grades depends on their instructor’s gender: When students get the grades they expected, male instructors get rewarded with better scores, when their expectations are not met, men get punished less than women. The bias is so strong that for young (under 35 years old) women teaching in male-dominated subjects, this has been shown to have an effect of up to 37% lower ratings for women.
These biases in student evaluations result in strengthening the position of an already privileged group: white, able-bodied, heterosexual, men of a certain age (ca 35-50 years old), who the students believe to be heterosexual and who are teaching in their (and their students’) first language get evaluated a lot more favourable than anybody who does not meet one or several of the criteria.
Sometimes evaluations are also used by students to express anger or frustration, and this can lead to abusive comments. Those comments are not distributed equally between all instructors, though, they are a lot more likely to be directed at women and other minorities, and they are cummulative. The more minority characteristics an instructor shows, the more abusive comments they will receive. This racist, sexist, ageist, homophobic abuse is obviously hurtful and harmful to an already disadvantaged population.
Reading the article, I can’t say I was surprised by the findings — unfortunately my impression of the general literature landscape on the matter was only confirmed by this systematic analysis. However, I was positively surprised to read the very direct way in which problematic aspects are called out in many places: “For example, women receive abusive comments, and academics of colour receive abusive comments, thus, a woman of colour is more likely to receive abuse because of her gender and her skin colour“. This is really disheartening to read on the one hand because it becomes so tangible and real, especially since in addition to being harmful to instructors’ mental health and well-being when they contain abuse, student evaluations are also still an important tool in determining people’s careers via hiring and promotion decisions. But on the other hand it really drives home the message and call to action to change these practices, which I really appreciate very much: “These practices not only harm the sector’s women and most underrepresented and vulnerable, it cannot be denied that [student evaluations of teaching] also actively contribute to further marginalising the groups universities declare to protect and value in their workforces.”.
So let’s get going and change evaluation practices!
Heffernan, T. (2021). Sexism, racism, prejudice, and bias: a literature review and synthesis of research surrounding student evaluations of courses and teaching. Assessment & Evaluation in Higher Education, 1-11.
Student feedback has become a fixture in higher education. But even though it is important to hear student voices when evaluating teaching and thinking of ways to improve it, students aren’t perfect judges of what type of teaching leads to the most learning, so their feedback should not be taken onboard without critical reflection. In fact, there are many studies that investigate specific biases that show up in student evaluations of teaching. So in order to use student feedback to improve teaching (both on the individual level when we consider changing aspects of our classes based on student feedback, as well as at an institutional level when evaluating teachers for personnel decisions), we need to be aware of the biases that student evaluations of teaching come with.
While student satisfaction may contribute to teaching effectiveness, it is not itself teaching effectiveness. Students may be satisfied or dissatisfied with courses for reasons unrelated to learning outcomes – and not in the instructor’s control (e.g., the instructor’s gender).
Boring et al. (2016)
In the following, I am not presenting a coherent theory (and if you know of one please point me to it!), these are snippets of current literature on student evaluations of teaching, many of which I found referenced in this annotated literature review on student evaluations of teaching by Eva (2018). The aim of my blogpost is not to provide a comprehensive literature review, rather than pointing out that there is a huge body of literature that teachers and higher ed administrators should know exists somewhere out there, that they can draw upon when in doubt (and ideally even when not in doubt ;-)).
This is quite scary, so I thought it made sense to start out with this study. Ambady and Rosenthal (1993) found that silent videos shorter than 30 seconds, in some case as short as 6 seconds, significantly predicted global end-of-semester student evaluations of teachers. These are videos that do not even include a sound track. Let this sink in…
And let’s get this out of the way right away: When students are asked to judge teaching effectiveness, that answer does not measure actual teaching effectiveness.
Stark and Freishtat (2014) give “an evaluation of course evaluations”. They conclude that student evaluations of teaching, though providing valuable information about students’ experiences, do not measure teaching effictiveness. Instead, ratings are even negatively associated with direct measures of teaching effectiveness and are influenced by gender, ethnicity and attractiveness of the instructor.
Uttl et al. (2017) conducted a meta-analysis of faculty’s teaching effectiveness and found that “student evaluation of teaching ratings and student learning are not related”. They state that “institutions focused on student learning and career success may want to abandon [student evaluation of teaching] ratings as a measure of faculty’s teaching effectiveness”.
Nasser-Abu Alhija (2017) showed that out of five dimensions of teaching (goals to be achieved, long-term student development, teaching methods and characteristics, relationships with students, and assessment), students viewed the assessment dimension as most important and the long-term student development dimension as least important. To students, the grades that instructors assigned and the methods they used to do this were the main aspects in judging good teaching and good instructors. Which is fair enough — after all, good grades help students in the short term — but that’s also not what we usually think of when we think of “good teaching”.
Kornell and Hausman (2016) review recent studies and report that when learning is measured at the end of the respective course, the “best” teachers got the highest ratings, i.e. the ones where the students felt that they had learned the most (which is congruent with Nasser-Abu Alhija (2017)’s findings of what students value in teaching). But when learning was measured during later courses, i.e. when meaningful deep learning was considered, other teachers seem to have more effective. Introducing desirable difficulties is thus good for learning, but bad for student ratings.
Carpenter et al. (2013) compared a fluent video (instructor standing upright, maintaining eye contact, speaking fluidly without notes) and a disfluent video (instructor slumping, looking away, speaking haltingly with notes). They found that even though the amount of learning that took place when students watched either of the videos wasn’t influenced by the lecturer’s fluency or lack thereof, the disfluent lecturer was rated lower than the fluent lecturer.
The authors note that “Although fluency did not significantly affect test performance in the present study, it is possible that fluent presentations usually accompany high-quality content. Furthermore, disfluent presentations might indirectly impair learning by encouraging mind wandering, reduced class attendance, and a decrease in the perceived importance of the topic.”
When students rate teachers effectiveness, they do that based on their assumption of how effective a teacher should be, and it turns out that they have different expectations depending on the gender of their teachers. El-Alayi et al. (2018) found that “female professors experience more work demands and special favour requests, particularly from academically entitled students”. This was both true when male and female faculty reported on their experiences, as well as when students were asked what their expectations of fictional male and female teachers were.
Boring (2017) found that even when learning outcomes were the same for students in courses taught by male and female teachers, female teachers received worse ratings than male teachers. This got even worse when teachers didn’t act in accordance to the stereotypes associated with their gender.
MacNell et al. (2015) found that believing that an instructor was female (in a study of online teaching where male and female names were sometimes assigned according to the actual gender of the teacher and sometimes not) was sufficient to rate that person lower than an instructor that was believed (correctly or not) to be male.
This title was drawn from the abstract of Pittman (2010)’s article that I unfortunately didn’t have access to, but thought an important enough point to include anyway.
There are very many more studies on race, and especially women of color, in teaching contexts, which all show that they are facing a really unfair uphill battle.
Rubin and Smith (1990) investigated “effects of accent, ethnicity, and lecture topic on undergraduates’ perceptions of nonnative English-speaking teaching assistants” in North America and found that 40% of undergraduates avoid classes instructed by nonnative English-speaking teaching assistants, even though the actual accentedness of teaching assistants did not actually influence student learning outcomes. Nevertheless, students judged teaching assistants they perceived as speaking with a strong accent as poorer teachers.
Similarly, Sanchez and Khan (2016) found that “presence of an instructor accent […] does not impact learning, but does cause learners to rate the instructor as less effective”.
Ewing et al. (2003) report that lecturers that were identified as gay or lesbian received lower teaching ratings than other lecturers with undisclosed sexual orientation when they, according to other measures, were perfoming very well. Poor teaching performance was, however, rated more positively, possibly to avoid discriminating against openly gay or lesbian lecturers.
Stonebraker and Stone (2015) find that “age does affect teaching effectiveness, at least as perceived by students. Age has a negative impact on student ratings of faculty members that is robust across genders, groups of academic disciplines and types of institutions”. Apparently, when it comes to students, from your mid-40ies on, you aren’t an effective teacher any more (unless you are still “hot” and “easy”).
Boring et al. (2016) find that “[student evaluation of teaching] are more sensitive to students’ gender bias and grade expectations than they are to teaching effectiveness.“
Pay attention to student comments but understand their limitations. Students typically are not well situated to evaluate pedagogy.
Stark and Freishtat (2014)
Does all of the above mean that student evaluations are biased in so many ways that we can’t actually learn anything from them? I do think that there are things that should not be done on the basis of student evaluations (e.g. rank teacher performance), and I do think that most times, student evaluations of teaching should be taken with a pinch of salt. But there are still ways in which the information gathered is useful.
Even though student satisfaction is not the same as teaching effectiveness, it might still be desirable to know how satisfied students are with specific aspects of a course. And especially open formats like for example the “continue, start, stop” method are great for gaining a new perspective on the classes we teach and potentially gaining fresh ideas of how to change things up.
Also tracking ones own evaluation over time is helpful since — apart from aging — other changes are hopefully intentional and can thus tell us something about our own development, at least assuming that different student cohorts evaluate teaching performance in a similar way. Also getting student feedback at a later date might be helpful, sometimes students only realize later which teachers they learnt from the most or what methods were actually helpful rather than just annoying.
A measure that doesn’t come directly from student evaluations of teaching but that I find very important to track is student success in later courses. Especially when that isn’t measured in a single grade, but when instructors come together and discuss how students are doing in tasks that build on previous courses. Having a well-designed curriculum and a very good idea of what ideas translate from one class to the next is obviously very important.
It is also important to keep in mind that, as Stark and Freishtat (2014) point out, statistical methods are only valid if there are enough responses to actually do statistics on them. So don’t take very few horrible comments to heart and ignore the whole bunch of people who are gushing about how awesome your teaching is!
P.S.: If you are an administrator or on an evaluation committee and would like to use student evaluations of teaching, the article by Linse (2017) might be helpful. They give specific advice on how to use student evaluations both in decision making as well as when talking to the teachers whose evaluations ended up on your desk.
Ambady, N., & Rosenthal, R. (1993). Half a minute: Predicting teacher evaluations from thin slices of nonverbal behavior and physical attractiveness. Journal of Personality and Social Psychology, 64(3), 431–441. https://doi.org/10.1037/0022-3514.64.3.431
Boring, A. (2017). Gender biases in student evaluations of teachers. Journal of Public Economics, 145(13), 27–41. https://doi.org/10.1016/j.jpubeco.2016.11.006
Boring, A., Dial, U. M. R., Ottoboni, K., & Stark, P. B. (2016). Student evaluations of teaching (mostly) do not measure teaching effectiveness. ScienceOpen Research, (January), 1–36. https://doi.org/10.14293/S2199-1006.1.SOR-EDU.AETBZC.v1
Carpenter, S. K., Wilford, M. M., Kornell, N., & Mullaney, K. M. (2013). Appearances can be deceiving: Instructor fluency increases perceptions of learning without increasing actual learning. Psychonomic Bulletin & Review, 20(6), 1350–1356. https://doi.org/10.3758/s13423-013-0442-z
El-Alayi, A., Hansen-Brown, A. A., & Ceynar, M. (2018). Dancing backward in high heels: Female professors experience more work demands and special favour requests, particularly from academically entitled students. Sex Roles. https://doi.org/10.1007/s11199-017-0872-6
Eva, N. (2018), Annotated literature review: student evaluations of teaching (SET), https://hdl.handle.net/10133/5089
Ewing, V. L., Stukas, A. A. J., & Sheehan, E. P. (2003). Student prejudice against gay male and lesbian lecturers. Journal of Social Psychology, 143(5), 569–579. http://web.csulb.edu/~djorgens/ewing.pdf
Kornell, N. & Hausman, H. (2016). Do the Best Teachers Get the Best Ratings? Front. Psychol. 7:570. https://doi.org/10.3389/fpsyg.2016.00570
Linse, A. R. (2017). Interpreting and using student ratings data: Guidance for faculty serving as administrators and on evaluation committees. Studies in Educational Evaluation, 54, 94- 106. https://doi.org/10.1016/j.stueduc.2016.12.004
MacNell, L., Driscoll, A., & Hunt, A. N. (2015). What’s in a name: Exposing gender bias in student ratings of teaching. Innovative Higher Education, 40(4), 291– 303. https://doi.org/10.1007/s10755-014-9313-4
Nasser-Abu Alhija, F. (2017). Teaching in higher education: Good teaching through students’ lens. Studies in Educational Evaluation, 54, 4-12. https://doi.org/10.1016/j.stueduc.2016.10.006
Pittman, C. T. (2010). Race and Gender Oppression in the Classroom: The Experiences of Women Faculty of Color with White Male Students. Teaching Sociology, 38(3), 183–196. https://doi.org/10.1177/0092055X10370120
Rubin, D. L., & Smith, K. A. (1990). Effects of accent, ethnicity, and lecture topic on undergraduates’ perceptions of nonnative English-speaking teaching assistants. International Journal of Intercultural Relations, 14, 337–353. https://doi.org/10.1016/0147-1767(90)90019-S
Sanchez, C. A., & Khan, S. (2016). Instructor accents in online education and their effect on learning and attitudes. Journal of Computer Assisted Learning, 32, 494–502. https://doi.org/10.1111/jcal.12149
Stark, P. B., & Freishtat, R. (2014). An Evaluation of Course Evaluations. ScienceOpen, 1–26. https://doi.org/10.14293/S2199-1006.1.SOR-EDU.AOFRQA.v1
Stonebraker, R. J., & Stone, G. S. (2015). Too old to teach? The effect of age on college and university professors. Research in Higher Education, 56(8), 793–812. https://doi.org/10.1007/s11162-015-9374-y
Uttl, B., White, C. A., & Gonzalez, D. W. (2017). Meta-analysis of faculty’s teaching effectiveness: Student evaluation of teaching ratings and student learning are not related. Studies in Educational Evaluation, 54, 22-42. http://dx.doi.org/10.1016/j.stueduc.2016.08.007
How do we measure whether teaching interventions really do what they are supposed to be doing? (Spoiler alert: In this post, I won’t actually give a definite answer to that question, I am only talking about a paper I read that I found very helpful, and reflecting on a couple of ideas I am currently pondering. So continue reading, but don’t expect me to answer this question for you! :-))
As I’ve talked about before, we are currently working on a project where undergraduate mathematics and mechanics teaching are linked via online practice problems. Now that we are implementing this project, it would be very nice to have some sort of “proof” of its effectiveness.
My (personal) problem with control group studies
Control group studies are likely the most common way to “scientifically” determine whether a teaching intervention had the desired effect. This has rubbed me the wrong way for some time — if I am so convinced that I am improving things, how can I keep my new and improved course from half of the students that I am working to serve? Could I really live with myself if we, for example, measured that half of the students in the control group dropped out within the first three or four weeks of our undergraduate mathematics course, while of the experimental group, only much fewer students dropped out, and much later in the semester? On the other hand, if our intervention had such a large effect, shouldn’t we be measuring it (at least once) in a classical control group study, so we know for sure what its effect is, in order to convince stakeholders at our and other universities that our intervention should be adopted everywhere? If the intervention really improves this much, everybody should see the most compelling evidence so that everybody starts adopting the intervention, right?
A helpful article
Looking for answers to the questions above, I asked Nicki for help, and she pointed me to a presentation by Nick Tilley (2000), that I found really eye-opening and helpful for framing those questions differently, and starting to find answers. The presentation is about evaluation in a social sciences context, but easily transferable to education research.
In this presentation, Tilley first places the proposed method of “realistic evaluation” in the larger context of philosophy of science. For example Popper (1945) suggests using small-scale interventions to deal with specific problems instead of large interventions that address everything at once, and points to the opportunities to investigate the extent to which the theories (on which those small-scale interventions were built) can be tested and improved. Similarly, Campbell (1999) talks about “reforms as experiments”. So the “realistic evaluation” paradigm has been around for a while, partly in conflict with how we do science “conventionally”.
Reality is too complex for control group studies
Then, Tilley talks about classical methods, specifically control group experiments, and argues that — in contrast to what is portrayed in washing detergent ads, for example — studys are typically too complex to directly transfer results between different contexts. In contrast to what science typically does, we are also not investigating a law of nature, where the goal is to understand a mechanism causing a regularity in a given context. Rather, we are investigating how we can cause a change in a regularity. This means we are asking the question “what works for whom in what circumstances?”. With our intervention, we might be introducing different mechanisms, triggering a change in balance of several mechanisms, and hence change the regularities under investigation (which, btw, is our goal!) — all by changing the context.
The approach for evaluations of interventions should therefore, according to Tilley, be “Context Mechanism Outcome Configurations” (CMOC), which describe the interactions between context, mechanism and outcome. In order to create such a description, one needs to clearly describe the mechanisms (“what is it about a measure which may lead it to have a particular outcome pattern in a given context?”), context (“what conditions are needed for a measure to trigger mechanisms to produce particular outcome patterns?”), outcome pattern (“what are the practical effects produced by causal mechanisms being triggered in a given context?” and this finally leads to CMOCs (“How are changes in regularity (outcomes) produced by measures introduced to modify the context and balance of mechanisms triggered?”).
Impact of CCTV on car crimes — a perfect example for control group studies?
Tilley gives a great example for how this works. Investigating how CCTV affects rates of car crimes seems to be easily measured by a classical control group setup. Just install the cameras and compare their crime rates with those of parking spaces without cameras! However, once you start thinking about mechanisms through which the CCTV cameras could influence crime rates, there are lots of different possible mechanisms. There are eight named explicitly in the presentation, for example offenders could be caught thanks to CCTV and go to jail, hence crime rates would sink. Or, criminals might not choose to commit crimes, because the risk of being caught increased due to CCTV, which would again result in lower crime rates. Or people using the car park might feel more secure in using it and therefore start using it more, making it busier at previously less busy times, making car theft more difficult and risky, leading to sinking crime rates.
But then, we also need to think about context, and how car parks and car park crimes potentially differ. For example, crime rate can be the same whether there are a few very active criminals, or many not as busy ones. So catching the similar number of offenders might have a different effect, depending on context. Or the pattern of usage of car parks might depend on working hours of people working close by. So if the dominant CCTV mechanism would be to increase confidence in usage, this would not really help because the busy hours are dedicated by people’s schedules, not how safe they feel. If this would lead to higher usage, however, more cars being around might mean more car crimes because there are more opportunities, yet still a decreased crime rate per use. Another context would be that thieves might just look for new targets outside of the one car park that is now equipped with CCTV, thereby just displacing the problem elsewhere. And there are a couple more contexts mentioned in the presentation.
Long story short: Even for a relatively simple problem (“how does CCTV affect car crime rate?”), there is a wide range of mechanisms and contexts which will all have some sort of influence. Just investigating one car park with CCTV and a second one without will likely not lead to results that help solve the car crime issue once and for all everywhere. First, theories of what exactly the mechanisms and contexts are for a given situation need to be developed, and then other methods of investigation are needed to figure out what exactly is important in any given situation. Do people leave their purses sitting out visibly in the same way everywhere? How are CCTV cameras positioned relative to the cars being stolen? Are usage pattern the same in two car parks? All of this and more needs to be addressed to sort out which of the context-mechanism theories above might be dominant at any given car park.
Back to mathematics learning and our teaching intervention
Let’s get back to my initial question that, btw, is a lot more complex than the example given in the Tilley-presentation. How can we know whether our teaching intervention is actually improving anything?
Mechanisms at play
First, let’s think about possible mechanisms at play here. “What is it about a measure which may lead it to have a particular outcome pattern in a given context?” Without claiming that this is a comprehensive list, here are a couple of ideas:
a) students might realize that they need mathematics to work on mechanics problems, increasing their motivation to learn mathematics
b) students might have more opportunity to receive feedback than before (because now the feedback is automated), and more feedback might lead to better learning
c) students might appreciate the effort made by the instructors, feel more valued and taken seriously, and therefore be more motivated to put in effort
d) students might prefer the online setting over classical settings and therefore practice more
e) students might have more opportunity to practice because of the flexibility in space and time given by the online setting, leading to more learning
f) students might want to earn the bonus points they receive for working on the practice problems
g) students might find it easier to learn mathematics and mechanics because they are presented in a clearer structure than before
Contexts
Now contexts. “What conditions are needed for a measure to trigger mechanisms to produce particular outcome patterns?” Are all students and all student difficulties with mathematics the same? (Again, this is just a spontaneous brain storm, this list is nowhere near comprehensive!)
– if students’ motivation to learn mathematics increased because they see that they will need it for other subjects (a), this might lead to them only learning those topics where we manage to convey that they really really need them, and neglecting all the topics that might be equally important but where we, for whatever reasons, just didn’t give as convincing an example
– if students really value feedback this highly (b), this might work really well, or there might be better ways to give personalised feedback
– if students react to feeling more valued by the instructor (c), this might only work for the students who directly experienced a before/after when the intervention was first introduced. As soon as the intervention has become old news, future cohorts won’t show the same reaction any more. It might also only work in a context where students typically don’t feel as valued so that this intervention sticks out
– if students prefer the online setting over classical settings generally (d), or appreciate the flexibility (e), this might work for us while we are one of the few courses offering such an online setting. But once other courses start using similar settings, we might be competing with others, and students might spend less time with us and our practice problems again
– if students mainly work for the bonus points (f), their learning might not be as sustainable as if they were intrinsically motivated. And as soon as there are no more bonus points to be gained, they might stop using any opportunity for practice just for practice’s sake
– providing students a structure (g) might make them depend on it, harming their future learning (see my post on this Teufelskreis).
Outcome pattern
Next, we look at outcome patterns: “what are the practical effects produced by causal mechanisms being triggered in a given context?”. So which of the mechanisms identified above (and possibly others) seem to be at play in our case, and how do they balance each other? For this, we clearly need a different method than “just” measuring the learning gain in an experimental group and compare it to a control group. We need a way to identify the mechanisms at play in our case, and those that are not. We then need to figure out the balance of those mechanisms. Is the increased interest in mathematics more important than students potentially being put off by the online setting? Or is the online setting so appealing that it compensates for the lack of interest in mathematics? Can we show students that we care about them without rolling out new interventions every semester, and will that motivate them to work with us? Do we really need to show the practical application of every tiny piece of mathematics in order for students to want to learn it, or can we make them trust us that we are only teaching what they will need, even if they aren’t yet able to see what they will need it for?
This is where I am currently at. Any ideas of how to proceed?
CMOCs
And finally, we have reached the CMOCs (“How are changes in regularity (outcomes) produced by measures introduced to modify the context and balance of mechanisms triggered?”). Assuming we have identified the outcome patterns, we would need to figure out how to change those outcome patterns, either by changing the context, or by changing the balance of mechanisms being triggered.
After reading this article and applying the concept to my project (and I only read the article today, so my thoughts will hopefully evolve some over the next couple of weeks!), I feel that the control group study that everybody seems to expect from us is not as valid as most people might think. As I said above, I don’t have a good answer yet for what we should do instead. But I found it very eye-opening to think about evaluations in this way and am confident that we will figure it out eventually! Luckily we have only run a small-scale pilot at this point, and there is still some time before we start rolling out the full intervention.
What do you think? How should we proceed?